Introduction
Welcome back to Neuvik’s Artificial Intelligence (AI) Risk Series – a blog series where we break down emerging cybersecurity trends related to AI. In this blog, we discuss what makes AI risk different, exploring risk through the AI “technology stack”. Missed our first blog? Check out the fundamentals of AI Risk Management for considerations on how to build, implement and manage your AI tooling securely: https://neuvik.com/article/securing-artificial-intelligence-1-it-starts-with-risk/.
When it comes to Artificial Intelligence (AI), most companies have rushed to embrace this new technology. However, many companies – even those with otherwise strong cybersecurity and technology management programs – treat AI as though it falls outside traditional IT management. Many also believe that security is inherent to AI, overlooking unique vulnerabilities from its multifaceted attack surface.
Spoiler alert: AI is vulnerable, and risks exist throughout the AI “tech stack” – and, concerningly, within the underlying models themselves. In this blog, we explore differences in AI risk and unpack useful lessons from securing Software-as-a-Service tools that can be applied to AI technologies.
What is the AI “tech stack” and what makes it unique?
To understand AI risk, it’s important to understand and visualize the “tech stack” underlying these tools.
There are several key components to AI:
- AI-Integrated “applications” – commercial or in-house developed applications that build on Generative AI (GenAI); these applications often provide specialized functionality restricted to a specific set of use cases
- AI “systems” – i.e., AI technologies created by an AI “provider” that allow enable querying, feedback, and generation of output from Large Language Models (LLMs)
- LLMs – the models underlying all AI systems and applications; in many cases, the LLMs are built and maintained by the same AI “providers” who offer AI “systems”
In many cases, the AI “provider” (such as Google or Anthropic) will name the AI “system” and “LLM” the same thing – for example, Gemini is built on Gemini LLMs, and Claude is built on Claude LLM. However, risk exists across each level.
A visualization of this “tech stack” is below. Note that the LLM and AI Systems inherently overlap – and any applications built on top add another layer.
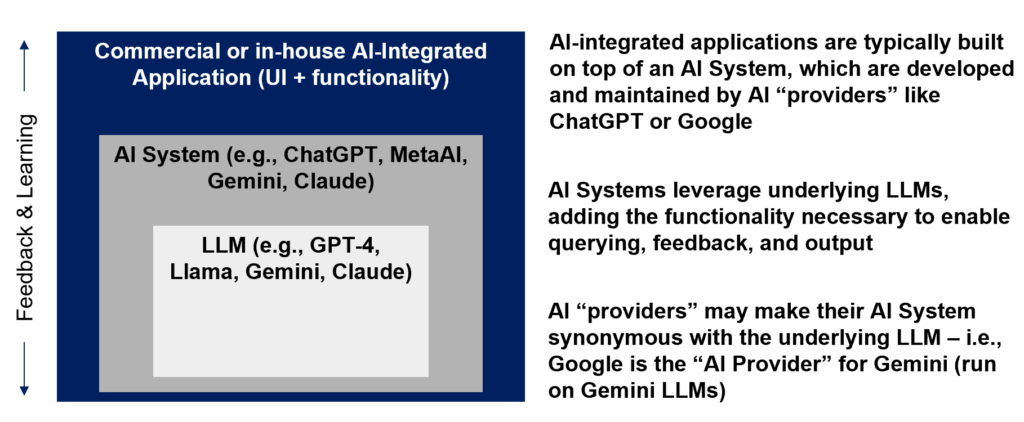
Seems Straightforward – Why is AI risk different?
The short answer: risk exists across all levels of the AI tech stack – but most organizations only consider risk at the application layer. As we’ll showcase throughout this blog series, risks exist at the “system” and “LLM” layers as well, and assuming providers have sufficiently implemented controls would be a mistake.
Most commercial GenAI applications, such as specialized chatbots and copilots, utilize an “AI System” (e.g., ChatGPT, Claude, Gemini) to facilitate querying of the underlying LLM (e.g., GPT-4, Claude, Gemini). Then, these commercial GenAI applications wrap that technology with a user-friendly customer interface. Finally, they implement “guardrails” limiting users to a specific set of use cases related to the application’s desired functionality (i.e., parsing health records, searching sales data).
Let’s illustrate this.
Consider a commercial chatbot (“SalesBot”) that a company purchased to perform sales-related customer support. The commercial application uses ChatGPT as the underlying AI “system” and was “trained” on the company’s sales data. This allows it to provide customers with a basic response to inquiries like, “What was my order total?” or “When will my contract renew?”
From an AI tech stack perspective, here’s how this maps back:
- Product Co 1 built “SalesBot”, the AI-integrated application
- “SalesBot” leverages ChatGPT as the underlying “AI System”
- ChatGPT leverages GPT-4 as the underlying “LLM”
While this seems straightforward, from the company’s perspective, they’re just purchasing SalesBot from Product Co 1. It seems intuitive that Product Co 1 would implement the security, right?
Spoiler #2: It’s never that simple.
Unlike traditional technology, companies overlook differences in AI risk
Now, let’s chat functionality. Product Co 1 built SalesBot to perform a specific set of services – i.e., sales customer support. To function, SalesBot requires the company’s customers to input a unique identifier (such as an order number or customer name) as a form of “access control” to the application. When functioning as expected, the SalesBot will treat these unique identifiers as guardrails. This allows it to only respond with information related to that specific customer, despite the AI “system” containing data for every customer in the company’s sales database. This approach constitutes an “application layer” control intended to prevent unauthorized access to customer data.
Most companies recognize the risk at this application layer. For example, a malicious actor could “brute force” the unique identifier to gain access to sales data for a transaction they didn’t make.
However, most companies overlook the possibility of a malicious actor manipulating the underlying AI “service” upon which SalesBot was built. A malicious actor could pose as a customer service representative, requiring access to the full sales data (we talk more about this type of manipulation – “prompt injection” – in Securing AI #4). Not only does this bypass the commercial guardrails, but is an attempt to manipulate the underlying GenAI system itself (which Product Co 1 likely didn’t restrict). Worse yet, a malicious actor might be able to “poison” the data SalesBot was trained on, causing it to spew incorrect data to customers. This is an example of risk at the LLM level, and – you guessed it – Product Co 1 likely can’t prevent this either.
As you can see, risk exists at each layer of the AI tech stack but could be invisible if companies expect commercial applications to provide sufficient security controls.
Of course, many organizations opt to build their own GenAI-integrated tools in-house, with the hope of reducing some of the associated risks with commercial products. However, many of these organizations fail to realize that the risks associated with the underlying GenAI service and LLMs remain, no matter who builds the “application” on top. Being mindful of these risks is critical.
So, what can we learn from cloud security and Software-as-a-Service tools?
Like the AI tech stack, Software-as-a-Service (SaaS), along with a variety of other cloud-related services such as “Platform-as-a-Service” and “Infrastructure-as-a-Service”, use integrations to stack technologies. At its simplest, SaaS essentially refers to an application build on top of a cloud provider (such as Amazon Web Services or Google Cloud Platform).
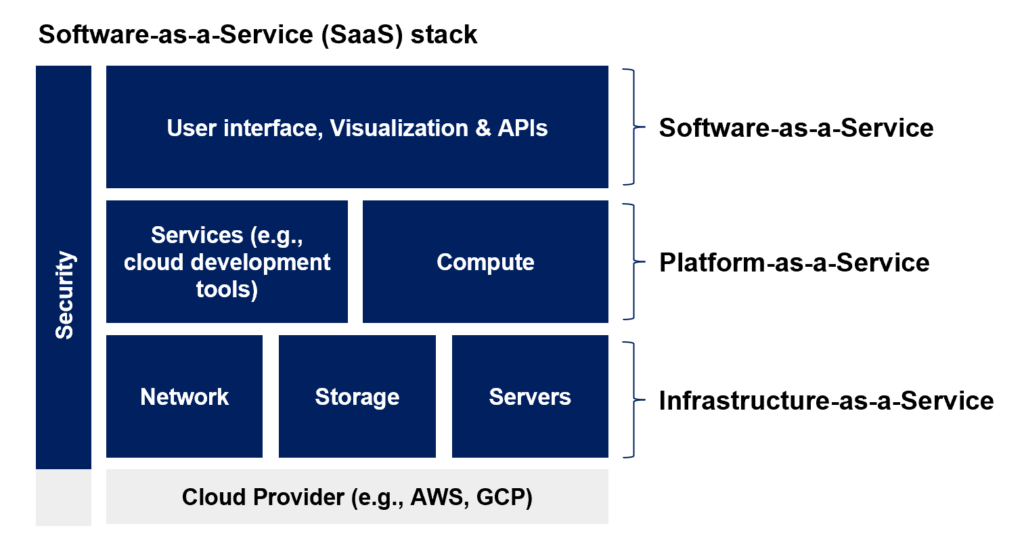
Given SaaS products have been in use for 20+ years, organizations now expect SaaS vendors (and the cloud providers underlying SaaS products) to provide not only application-layer security, but infrastructure- and data-layer security as well. However, this wasn’t always the case – and, even today, both SaaS vendors and cloud providers experience vulnerabilities.
Similarly, organizations using cloud providers like AWS or GCP to build their own applications (for commercial or in-house use) expect the service providers – Amazon, Microsoft, or Google – to provide appropriate security, maintenance, and uptime. While the company building the application can ensure the security of their own product.
So, what can we learn from cloud security and SaaS tooling?
By now, most organizations are familiar with risks associated with both SaaS applications and cloud providers and have robust programs to perform technical validation of security controls and proactively manage risk. These activities include but aren’t limited to requiring proof of compliance with technical security requirements, vendor risk assessments, cloud security assessments, application-specific penetration tests, configuration validations, access control validations, and more. Similarly, SaaS tools are incorporated into asset inventories and vendors are required to perform regular security updates and maintenance as part of standard IT operating procedure.
As with SaaS, AI applications should be held to the same standard. It’s imperative to evaluate risk, perform technical testing, and ensure robust AI vendor risk management.
Conclusion
While AI technologies may seem secure, organizations should be vigilant to avoid introducing risk. Cyber risk exists at all layers of the AI technology stack, and organizations can leverage lessons from SaaS and cloud technology to understand how to protect their data.
Want to protect your organization? Contact us today or learn more about Neuvik’s AI Risk Management services: https://neuvik.com/our-services/cyber-risk-management/.